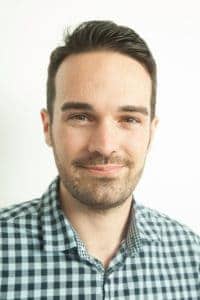
Ryan Kelly, vice president of marketing, Nanigans
It’s happened to all of us at one time or another. After a few days of consideration, you finally make that online purchase. Then you see ads for that product everywhere you go on the internet.
From the marketer’s perspective, it’s hard to think of a worse use of your advertising budget. The one person who’s not interested in buying your product is the consumer who just bought it.
This isn’t just annoying for marketers though. Some 88 percent of consumers are tired of seeing ads for products they already bought, according to recent survey by Nanigans among 1,000 U.S. customers ages 25-54. There are three main reasons why such ads keep happening. The first two are to some extent out of marketers’ control. However, the third—improving your recommendation engine—isn’t.
3 reasons for poor retargeting ads
Marketers don’t intentionally choose to keep running irrelevant ads, of course. The first reason they persist is that cross-device or cross-browser identification can still be inaccurate. In a 2017 survey, 86 percent of marketers said they were unable to accurately measure their target consumers’ cross-device activity. If you don’t recognize that Consumer X who bought something on her desktop is also Consumer X on her smartphone, then there’s a high likelihood you’ll show her the wrong ads.
Another factor is data delays. Even if a customer makes an online purchase, the retargeting recommendations often aren’t updated quickly enough. Many retargeting vendors do relatively infrequent server-to-server updates for data syncing. But even after syncing the data, the retargeting vendor’s systems may have data processing delays, model retraining times, and various queued services that add up to substantial recommendation delays. So if Consumer X buys khakis on JCrew.com, there will be enough of a recommendation lag that he’ll see an ad for the khakis he just bought (or another redundant or irrelevant ad) if he immediately visits CNN.com after the purchase.
The third reason is that a marketer is working with a retargeting vendor that’s employing an ineffective product recommendation engine. This is an area where marketers could have more control.
When recommendation engines go wrong
An exceptional product recommendation engine can be a huge asset for a brand. McKinsey estimates recommendation engines account for 35 percent of Amazon’s sales. Yet there are reasons why recommendation engines can go off the rails. A recent research paper explains that product recommendation suggestions fall into two categories: substitutes or complements.
If you’re browsing for a new smartphone, then an ad for a Samsung Galaxy S9 makes sense because you’re looking for a substitute for your current phone. But if you just purchased a phone, you’ll want complementary recommendations for products like a case or a wireless charging mat.
Unfortunately, not all recommendation engines are fine-tuned enough to comprehend the difference between substitutes and complements. And that’s when ads for red sneakers are served to someone who just bought the same sneakers in a different color.
There are other nuances as well. A 2015 paper by two Wharton professors found that because product recommendations are based on sales, recommendations don’t tend to surface “long-tail” items. Take for example UGG and their classic boots. The “long-tail” for UGG may be slippers or scarfs. However, the success of their iconic boots leads to a “rich get richer” phenomenon in which the recommendation engines push everyone more boots. Most of the product recommendation engines used by third-party retargeting vendors fail miserably at surfacing novel items. UGG likely doesn’t need help selling more boots, especially to consumers who just bought a pair.
Additionally, precise recommendations aren’t universal so the “one size fits all” approach that third-party vendors commonly use won’t work well. For instance, a good recommendation engine for mattress retailer Casper won’t make sense for Ikea because even though they both sell products online, the needs of their customers—how often they purchase, what products are substitutes versus complements—are vastly different.
Taking it in-house
As with many digital marketing tools, marketers can benefit from using their own recommendation engines rather than relying on a retargeting vendor’s engine.
Most online retailers already have a product recommendation engine. But even if they don’t, building one isn’t difficult. Open source tools like Apache Mahout or Spark can help you get up and running. A good starting point would be a collaborative filtering algorithm such as Singular Value Decomposition that will make recommendations purely based on which items are purchased or viewed together by the same users.
A custom-built model will have more finely tuned signals to understand customers’ purchasing behavior. For example, the wireless charging mat may be a good recommendation for someone who recently bought a phone, but an off-the-shelf recommendation engine will just as often recommend purchasing the phone for users who purchased the mat. A custom-built recommendation engine would deduce that the user likely already owns that specific phone and then offer a better recommendation.
All that said, the sad fact is most third-party retargeting vendors won’t allow you to use your own recommendation engine even if you have one.
If you ask yourself why that is, it’s because their system is rigged in their own favor at the expense of your brand. And until marketers demand more from their “partners”, consumers will continue to see irrelevant retargeting ads.
Nanigans provides cross-channel advertising software.
Favorite