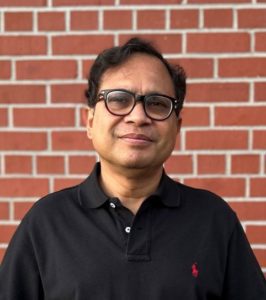
Prosenjit Sen
In my first article in this series, What B2B must learn from B2C, I discussed how, despite the huge promise of B2B commerce, this space faces significant challenges for both pre-sales and post-sales due to limitations of existing technologies like “search.” In the second article, I discussed how you can Skyrocket product discovery on your B2B site using the latest AI/ML technologies. In this article I will discuss how you can provide top-tier post-sales support to keep customers happy, at a low cost.
Today, for post-sales you probably have omnichannel Level 1 and Level 2 support where your support staff chat with customers live or speak with them over the phone to understand their issue, and then look up the answers from your reference documents to help the customer.
Often, if the issue is complex, they create a ticket and pass it on to Level 3 support. For such complex issues, a lot of time gets wasted with L1 or L2 support trying to investigate the issue first, before escalating.
Technologies to Consider
In my last article. on product search, I discussed some of the latest technological advances in deep learning, natural language processing (NLP) and computer vision (CV). With such technologies, it is now possible to back-end a chatbot or voice-bot with instant answers directly from your reference documents, thus taking self-service to the next level, and making it a viable tool for post-sales support.
Chatbots have traditionally used a pre-defined decision tree for guiding a conversation. They also had the limitation of relying on data like FAQs or logs. This technology has now evolved where you can have an “unbounded conversation” with the user, using answers directly from a company’s reference documents. Such Chatbot 2.0’s can be used to automatically handle a significant portion of support issues.
Voice bot technology is also improving; however, even today, converting live voice (telephony) to text is often erroneous. But recent improvements in this space, can give you around 80% – 90% accuracy if the AI models for such conversion are trained with your voice data. This accuracy level is a very hopeful sign. Soon, it will be possible to interpret a live conversation between the customer and support staff, identify what the customer is asking for, and automatically pull up answers from your reference documents or from your back-end systems, such as ERP ordering systems.
Another self-service can be to automatically interpret a customer’s email or ticket on a support issue to identify the problem discussed, pull up answers from reference documents or back-end systems, and then automatically respond to the customer if the confidence in the responses is high. Again, deep learning and NLP now make this possible.
Use Cases
After-market purchase
Once a customer has purchased your product (air conditioners, heavy machinery, networking hardware, etc.), they need to be able to easily purchase after-market parts and tools using self-service. If you have the parts and tools data on your website, you should allow customers to converse with a chatbot to specify their exact specs/requirements and get information on features, compliance, etc. — And if you also provide a quick path to purchase, —this strategy will help drive sales and make customers loyal and happy.
The technology for this strategy involves the ability to get answers from structured, unstructured, and real-time reference data; I discussed this in my previous article on product discovery, Use Cases 1 and 2.
Customer has questions on installation and configuration
Instead of L1 or L2 support staff spending time on these queries, it is recommended that you use a chatbot or voice-bot based self-service. Please refer to my previous article, Use Case 3.
Customer submits a support case for a complex issue (in your Salesforce or ServiceNow system)
For complex products, often there will be complex issues for which a customer needs help rapidly. They would typically create a support ticket in Salesforce or ServiceNow that would go to your support queue for the right support engineer to work on it. The support engineer would typically open the ticket, read it and then submit a query using “search tools,” receive several documents, then open each document and read it. They do this repeatedly until they find the right solution. Then they may need to troubleshoot or reproduce the issue.
This is an inefficient and time-consuming process, because of which many customers’ tickets often go unattended. Using the technologies I discussed, you can automatically interpret a customer’s ticket to identify the issue topics discussed, then get the right answers from the reference documents so when the support agent opens a ticket (in Salesforce or ServiceNow), they see the answers (specific sections) from different documents already pulled up for them. This skyrockets their productivity, resulting in reduced MTTR and a reduction in unresolved tickets. As a result, you will have happy customers and ensure their loyalty. (MTTR stands for “mean time to resolve” customer service incidents.)
Help with Order Processing
Post-sale, your customers will occasionally need to know the status of their order, its estimated arrival, or to change or cancel the order. They may also need to see if certain other products associated with their purchase order are in stock or can be bundled in with the order placed. All this information is probably present in your SAP or other ERP system. You can provide customers with a self-service tool (chatbot or even voice-bot) so they can manage their needs expediently; this is very important to keep customers loyal.
Email Marketing
It is also a good idea to develop a database of customers and prospects and then send them information on new product releases, personalized based on the products they have bought, and promotions. You can also have a “Technology” section in the email communications where you can give them insight into the latest technologies and developments in their areas of interest.
Summary
Use automation throughout the support lifecycle — pre-sales and post-sales (discussed here). Keep your customers happy and informed with accurate information when they are looking to make a purchase and even post-purchase. If you use the right AI technology, you can often do this without spending major money, and the ROI should be achievable within six months.
In the next article, I will discuss how to provide the quickest path to purchase on your site and where support is heading in the years to come, given the ongoing developments in AI/ML technologies.
About the Author:
Prosenjit Sen is a serial entrepreneur and currently the CEO of Quark.ai, an “autonomous support” platform that uses deep learning, natural language processing (NLP), and computer vision (CV) to bring automation to sales support and field support. He was previously employee No. 5 as part of the founding team of Informatica, a pioneer in online data integration technology. Prosenjit is a mentor for the Alchemist Accelerator and the Bay Area IIT Startups Accelerator. And he is the co-author of the book “RFID for Energy & Utility Industries.”
Sign up
Sign up for a complimentary subscription to Digital Commerce 360 B2B News, published 4x/week. It covers technology and business trends in the growing B2B ecommerce industry. Contact editor Paul Demery at [email protected] and follow him on Twitter @pdemery.
Favorite